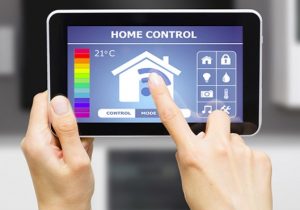
Nowadays manual climate control is widespread in residential, office and public spaces (opening/closing of the window, on/off heater), largely due to the low prevalence of automatic control devices. Public spaces claim high demands on air quality (e.g. hospitals, kindergartens), energy efficiency (hypermarkets, shopping malls) and are equipped with the necessary sensors and devices, but usually all devices are controlled independently. Smart climate devices are gradually entering the mass market and gaining popularity. Moreover, progressive construction companies are declaring energy efficiency and advanced climate management capabilities in their buildings. The construction of an integrated climate management system is hampered by the lack of an adequate mathematical model and/or lack of data from existing sensors and poor signal processing. Existing building models are based on the solution of nonlinear heat-mass transfer equations (Navier-Stokes).
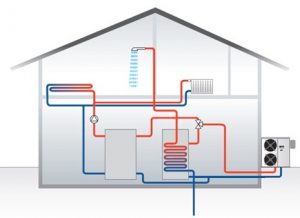
Analysis of the macro characteristics of air (average temperature, humidity, CO2 concentration) in complex multizone rooms requires simplified models to describe the air characteristics’ dynamics on a minute scale.
Project objectives:
- Building an advanced model of indoor air performance dynamics.
- Development of algorithms for identification of the constructed model on the basis of incomplete measurements.
- Development of algorithms for optimal control of microclimate formation devices, search for optimal operating modes taking into account the balance of group comfort and energy saving.
- Analysis of different operating modes taking into account the possibilities of distributed generation and consumption shift.
- Implementation of the developed control algorithms on the basis of the control polygon in the electric power industry of the Institute of Power Engineering of the Russian Academy of Sciences.
Approaches and methods of research: Optimal control, control methods with predictive model (MPC), methods of machine learning, distributed optimization.
Prospects: The developed algorithms of climate control can be used in the lower level models in the problem of forecasting the energy consumption of office buildings.